Remember pagers? Those little beeping devices that were the height of mobile communication back in the 80s and 90s? Well, they are still a mainstay in many hospitals today. As outdated as it seems, the trusty old pager has stuck around for their reliability and limited interruptions.
So when doctors at San Francisco General tried replacing pagers with texting, problems arose. Texting made contacting colleagues too easy. Doctors got interrupted constantly with non-urgent questions and chatter. The old pagers had an unexpected benefit - they forced doctors to be succinct and selective with their communication. Many doctors reverted to pagers. Similarly, transitioning from paper to electronic health records (EHRs) was painfully slow, especially with older physicians set in their ways. Thoughtful change management helped ease adoption.
These examples highlight a common challenge when new technologies are introduced in healthcare: adoption depends heavily on human factors like workflow, habits, and communication culture. As AI capabilities spread, from algorithms to virtual assistants, human-centered design is essential for successful integration. In fact, a recent survey showed that most clinicians have little understanding of how AI technologies actually work. Without engaging end users, even the most advanced AI risks rejection.
So how can we design AI solutions that providers and patients will embrace?
The Pitfalls of Technology-First Healthcare AI
The need for consumer centricity in healthcare is often spoken about, including this McKinsey article. And it got us thinking. Many early healthcare solutions (including AI) were created by technology companies without adequate collaboration with frontline healthcare professionals. This technology-first approach often fails to fully accommodate needs of both providers and patients and clinical workflows.
Let’s consider EHRs once again. These were mainly built for billing purposes, not to help doctors provide better care. The result? EHR systems that are painfully clunky and time-consuming for clinicians to use. Information overload. Hidden data. Endless popups and alerts. EHRs have become a huge driver of physician burnout, with over 50% of doctors now experiencing symptoms.
The lack of human-centered design in healthcare AI leads to problems like poor interfaces, AI recommendations that don't fit real-world contexts, propagation of biases, and potential negative consequences, emphasizing the need for AI to genuinely aid patients and doctors beyond just diagnostic accuracy.

This lack of human-centered design in healthcare AI development can lead to various pitfalls:
- Firstly, the obvious — Poor user interfaces that are confusing, cluttered, or add friction to workflows. Most tools end up clashing with the way healthcare providers work every day, adding to their stress.
- AI recommendations that fail to account for the nuances and uncertainties inherent in real clinical contexts. Black box algorithms that lack explainability can seem unreliable to clinicians.
- Propagation of biases that are present in clinical practice data used to train algorithms. For example, one prominent hospital algorithm favored white patients simply because more had been spent on their care historically. Cognitive biases like automation bias result in clinicians overly relying on AI recommendations without critical evaluation.
- Potential for unintended negative consequences like dataset shift, accidental fitting of confounders, or susceptibility to adversarial attacks. Continuous auditing and monitoring are required to detect issues before they veer systems off course.
- Once you move beyond accuracy, it's not just about getting the diagnosis right. It's about how AI actually helps patients and doctors in real life, which isn't always captured in the stats.
The design and implementation of healthcare AI must remain vigilant against these dangers through extensive user testing, auditing for biases, and centering patient perspectives.
A New Hope for Healthcare
Thus far, we've seen how technology-first approaches to healthcare AI can falter. So what's the antidote? Design thinking. But what exactly does design thinking and human-centered AI in healthcare mean? What core principles should guide its development and deployment?
For healthcare AI, a design thinking approach means assembling cross-functional teams of patients, clinicians, and administrators to ideate solutions. It is about keeping end user needs and perspectives at the heart of the design process. It means designing AI to enhance clinical practice rather than replace human judgment. And it means instilling patient priorities like privacy, trust, and transparency throughout the system lifecycle. Some key principles include:
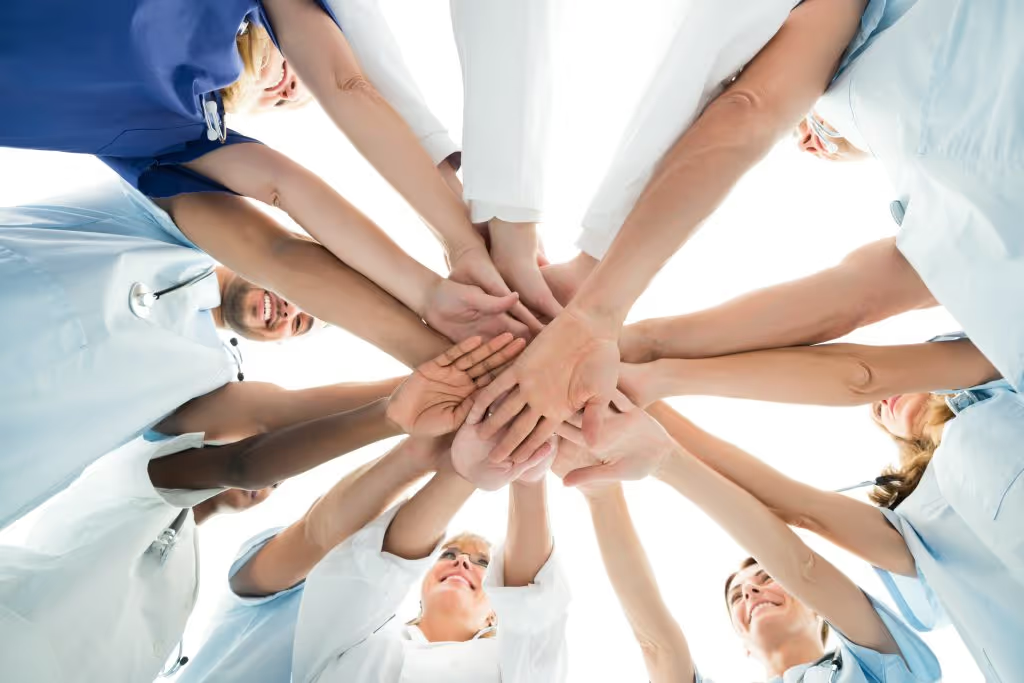
- Inclusive Design Teams: Patients, clinicians, administrators should all have a seat at the table. Cross-functional teams allow diverse perspectives to shape solutions. For example, AI systems in radiology have generally been more successful since they were developed in partnership with healthcare professionals, leading to tools that optimize patient scheduling and assist in diagnostic processes.
- AI to Enhance, Not Replace: Systems should aim to augment human skills and judgement, not replace them. Clinicians should retain agency over critical decisions.
- Patient Priorities: Patient data privacy, trust, and transparency should be top priorities. Allow patients to guide AI use for their care.
- Workflow Integration: Design AI tools to integrate smoothly into clinical and operational workflows. Avoid disrupting existing processes.
- Iterative Improvement: Regularly gather user feedback post-deployment to incrementally refine AI systems, ensuring they adapt to evolving needs.
While these provide high-level guidance, effectively applying human-centered principles requires getting into the nuances. Cross-functional teams must watch out for edge cases as they design. For example:
- How will AI change communication practices and culture? More asynchronous coordination?
- How to balance standardization vs. customization for diverse patients?
- How to account for complex comorbidities, inconsistencies in data, rare diseases?
- How might workflows and user needs evolve over time as AI integrates?
The Secret Ingredient of Human-Centered Design
A study by the CDC encompassing 24 projects found that design thinking in healthcare led to innovations that were more effective, efficient, and user-friendly compared to traditional approaches.
We know adoption and user trust is crucial for new technology. For instance, the Mayo Clinic's early adoption of design thinking in its Center for Innovation resulted in numerous small improvements, indicating that involving healthcare professionals in the design process can significantly increase their willingness to use and integrate AI technologies into their practice. Plus, we can avoid pitfalls from the get-go and create healthcare AI that actually helps patients and clinicians. How so? Well, design thinking is a bit like fast and furious brainstorming, prototyping, and testing with end users in the driver's seat. This means AI gets rapidly refined until it's something stakeholders truly want and need. Challenges around change management and training are often preempted because the cross-functional design team has already prepared stakeholders for workflow evolutions.
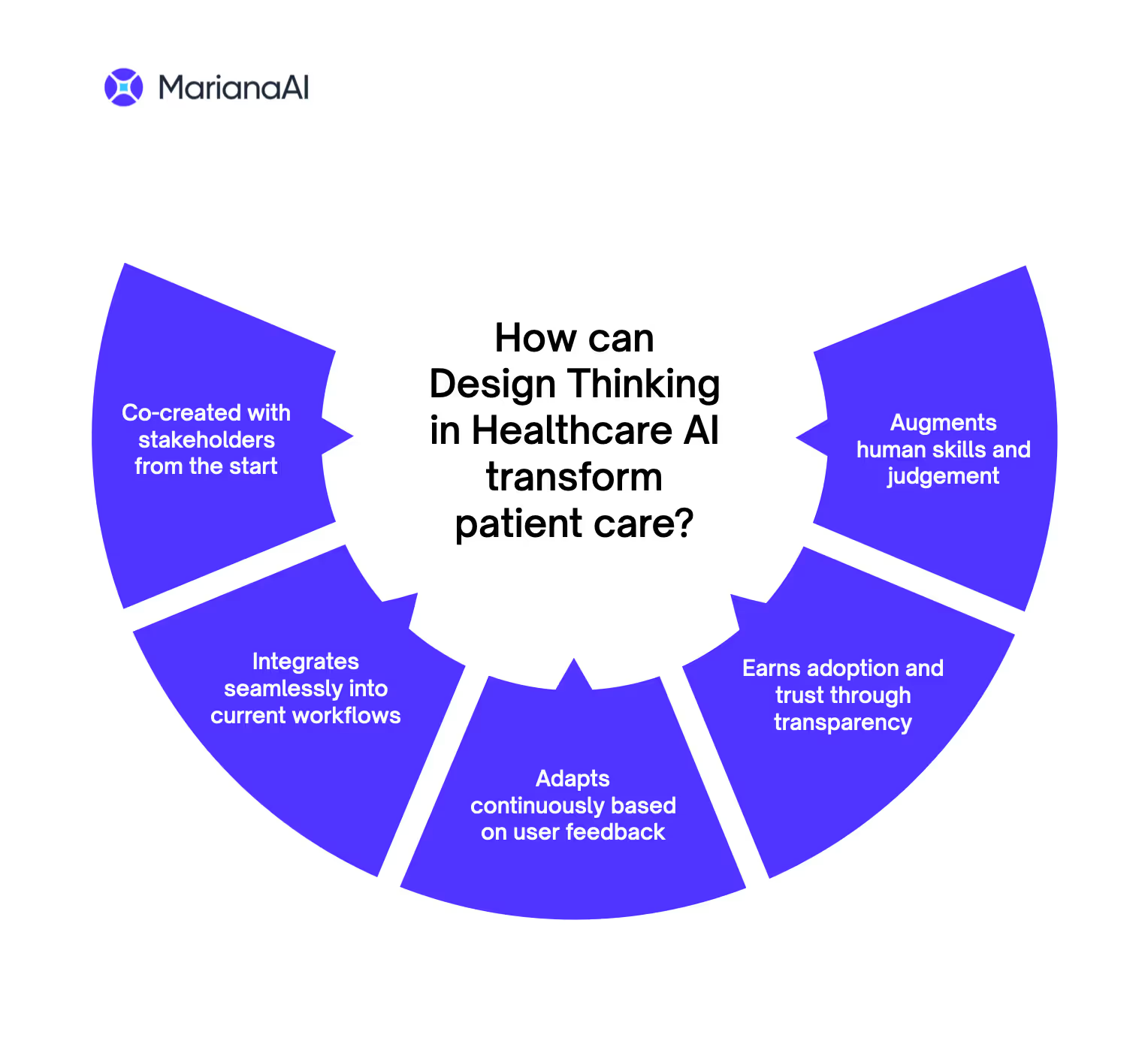
Design thinking also allows more personalization and customization for individual patients' needs, constraints, and preferences. And the collaborative, continuously iterating nature of the methodology significantly reduces long-term maintenance costs that might otherwise accrue from major required redesigns down the line. In essence, human-centered design provides a flexible process to create future-proof AI systems that can successfully scale and adapt as user needs change over time.
Bottom line, design thinking creates healthcare AI that works for people, not the other way around. Who wouldn't want that?
Building a Human-Centered Healthcare AI Assistant
So much about design thinking. Now, meet Dr. Sanchez. She's an experienced family medicine physician and has been one of our early adopters. With overloaded schedules and dozens of patients each day, Dr. Sanchez struggled to keep up with ever-evolving medical knowledge and deliver truly personalized care. Spent hours hunting down patient histories, piecing together care plans from multiple outdated records, and worrying she's missing critical details.
And she was not alone. Surveys showed over 50% of doctors were burned out (this has increased over the years and especially post-COVID), in large part due to clerical burdens and information overload. But what if AI could help? That's the idea behind MarianaGPT, a tool at the core of MarianaAI’s CARE platform. It was co-designed through an intensive design thinking process with real clinicians including Dr. Sanchez.
MarianaGPT integrates seamlessly with EHRs, instantly surfacing relevant patient histories so physicians need not waste time digging. It monitors treatment progress, flags discrepancies, and alerts to potential issues. Whenever you need medical knowledge or advice, you simply ask MarianaGPT. It scans millions of papers and clinical guidelines to summarize the latest evidence-based practices in seconds. Physicians can even leverage MarianaGPT to rapidly create personalized, precision care plans informed by each patient's unique health status and needs.
The result? Physicians like Dr. Sanchez feel empowered with the knowledge and time to focus on what matters most - building meaningful relationships and providing thoughtful care for her patients. And the best part is MarianaGPT keeps getting smarter with continual clinician feedback. It's AI designed for humans, by humans. Dr. Sanchez wishes all healthcare tools were this intuitive!
The Bottom Line
At the end of the day, the success of healthcare AI comes down to putting people first. The key reason it works is because it engages end users from start to finish. By assembling cross-disciplinary teams, observing clinical workflows, and co-designing solutions, hidden needs and constraints become clear. Rapid prototyping then allows ideas to be tested and refined quickly based on user feedback.
This human-centered process results in healthcare AI that feels intuitive rather than disruptive. Tools are tailored to enhance existing workflows, not complicate them. Just as importantly, involving stakeholders builds trust and ownership in the finished product.
Exciting opportunities lie ahead as this collaborative approach unlocks healthcare AI's immense potential. Areas like process automation, personalized medicine, and population health management are constantly looked into. In summary, by putting people first, AI becomes an invaluable asset rather than an liability. The human element matters now more than ever.